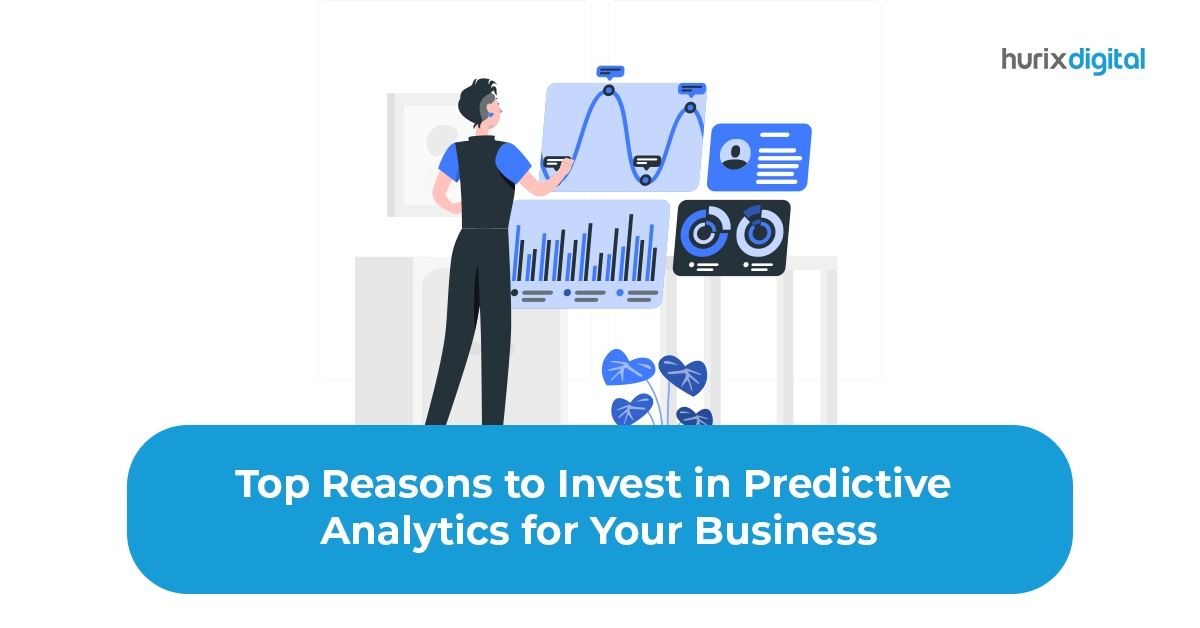
Top Reasons to Invest in Predictive Analytics for Your Business
Summary
A company can succeed or fail depending on its capacity to make wise decisions based on historical context. Right and wrong are very hard to separate at the rate at which businesses must expand. It can be worrisome when there does not seem to be a flat-out correct response during the decision-making process.
Businesses can learn a lot about their operations by employing sophisticated analytical techniques and collecting predictive data. As per Statista, for instance, 52% of marketing professionals applied AI tools to enhance operations and 51% to handle data.
This article will explore predictive data analytics and discuss the benefits it can bring to your business.
Table of Contents:
The Concept of Predictive Analytics
Predictive analytics predicts future outcomes based on current and historical data. It is applied to determine the probability of possible results, as opposed to conventional decision-making, which focuses on past events.
In several sectors, like retail and banking, this proactive strategy can greatly improve decision-making processes.
1. Components of Predictive Analytics
For precise and practical insights, predictive analytics depends on several essential elements:
- Data Collection: Data is the foundational element of predictive analytics. This covers historical, current, and even outside data sources that might affect the predictions. Businesses gather large volumes of data from a variety of sources, such as social media interactions and consumer transactions.
- Data Preprocessing: Cleaning and preprocessing the gathered data is necessary before analysis can start. Resolving missing values and organizing the data ensures that the input is accurate and ready for analysis.
- Feature Engineering: This stage selects and modifies the variables, also known as features, from the raw data. These variables will be used in the predictive model. Feature engineering is an important process that helps to choose the most relevant data.
- Model Selection and Training: Predictive analytics involves statistical and machine learning models, such as neural networks, decision trees, and regression analysis. The kind of data and the particular prediction work determine the model to be used. After being chosen, the model is trained with historical data to discover trends and connections in the material.
- Model Evaluation and Validation: To guarantee the correctness and dependability of the model, its performance is assessed after training with multiple methods. Methods like cross-validation and testing on untested data can evaluate how well the model generalizes to fresh data.
- Deployment and Monitoring: Following validation, a model is used to predict with fresh data. Since actual conditions and data can vary over time, constant monitoring and maintenance of the model are necessary to ensure its continued correctness and relevance.
2. Types of Predictive Analytics Models
In predictive analytics, several types of models are frequently used:
- Regression Models: These models are used for predicting continuous results. A simpler type is linear regression; more sophisticated models, such as logistic and polynomial regression, are employed to make deeper predictions.
- Classification Models: These models predict categorical results. Classification problems, such as estimating whether a consumer will purchase a product, frequently use techniques like decision trees, support vector machines, and neural networks.
- Time Series Models: These models look at time-aligned data. We can forecast future values by using exponential smoothing and ARIMA methods to analyze past data trends.
- Clustering Models: These are collections of related data elements. Data pattern identification commonly uses hierarchical and K-means clustering methods.
Also Read: How Business Analysis Consulting Services are Changing the Game
Benefits of Predictive Analytics
Predictive analytics offers your company the following benefits:
1. Improved Decision-Making
Making decisions traditionally depends on prior experiences. This method is not always dependable and prone to error. Predictive analytics is a data-driven method that reduces conjecture, in comparison.
Retailers can plan marketing campaigns and predict product demand and financial organizations can reduce the possibility of loan defaults by conducting precise credit risk assessments.
2. A Better Customer Experience
Through the analysis of consumer data to find patterns and trends, predictive analytics platforms let companies customize their products to satisfy their clients. Companies can promote the right products and customize marketing efforts.
eCommerce platforms can analyze customer purchase histories to suggest other items. This raises client loyalty while also enhancing the buying experience.
3. Optimized Operations
By finding inefficiencies and predicting possible problems before they happen, predictive analytics can help companies optimize their operations. Companies adopting this proactive approach can increase productivity and streamline operations.
Manufacturers can use predictive analytics to plan maintenance and anticipate equipment issues. This reduces maintenance and downtime expenses, ensuring smoother production.
Likewise, by forecasting weather and traffic patterns, logistical companies can optimize delivery routes and save on fuel.
4. Reduced Risks
Examining historical data helps businesses identify possible hazards and take preventative measures. Predictive analytics can help the banking sector spot irregularities in transaction patterns and illegal conduct. Insurance firms can more accurately evaluate policyholder risk to improve pricing and reduce the number of claims.
5. Enhanced Marketing Strategies
By examining consumer data and behavior, businesses can design focused and individualized marketing efforts that appeal to their target market. Companies can divide consumers according to different metrics and customize their messaging.
Predictive analytics can optimize email marketing campaigns by determining when emails should be sent and what kind of content appeals to different demographics. This customization leads to higher engagement rates and an improved ROI.
6. Improved Financial Performance
Using predictive data can help companies lower their operating costs and uncover additional revenue sources. Predictive analytics can also help finance businesses locate desirable clients and offer them specialized services. Merchants who wish to boost sales must know how customers react to prices.
Also Read: 10 Crucial Reasons to Hire a Business Analysis Consultant for Your Company
Conclusion
Predictive analytics is key to making your business as future-ready as possible. By utilizing its capabilities for making decisions, businesses can improve their bottom lines. With the business analysis service offered by Hurix Digital, you can collaborate with a team of experienced analysts to establish a strong foundation for your product development process.
So what are you waiting for? Contact us now to get started!
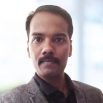
Currently serving as the Vice President of Technology Delivery Operations at HurixDigital, a prominent global provider of digital content and technology solutions for publishers, corporations, and educational institutions. With over 16 years of experience spanning EdTech and various domains, I hold certification as a SCRUM Product Owner (CSPO). My expertise includes operations, finance, and adept people management skills.