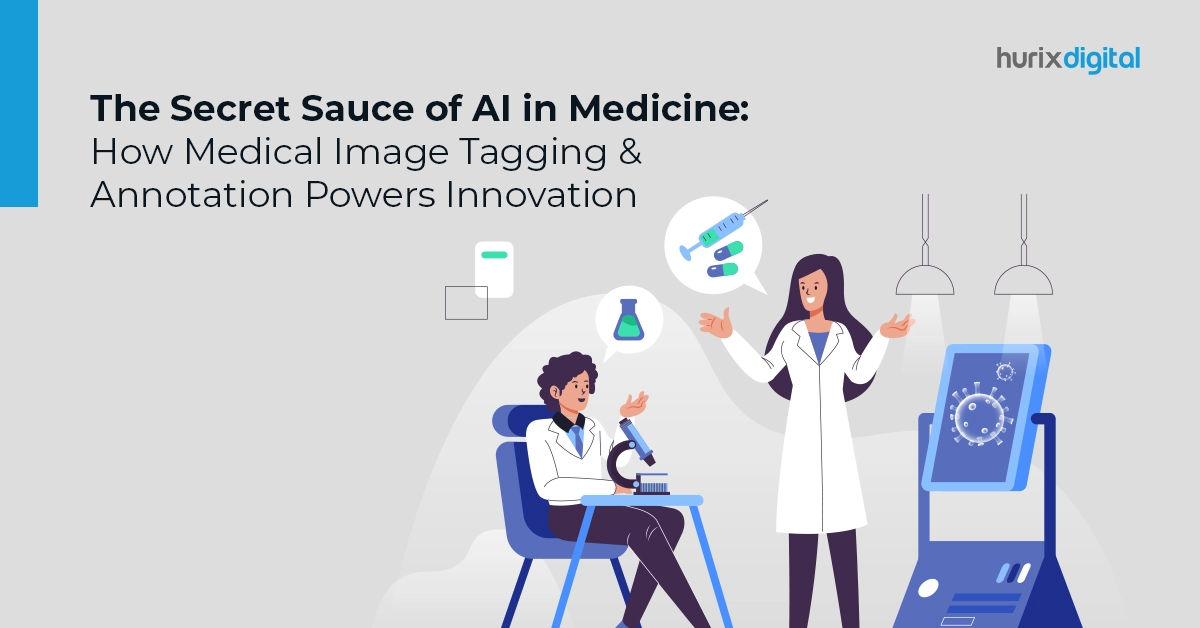
The Secret Sauce of AI in Medicine: How Medical Image Tagging & Annotation Powers Innovation
Summary
Explore how AI-driven medical image tagging and annotation are advancing innovation in medicine. This blog discusses the impact of AI on improving medical imaging and diagnostics.
Artificial intelligence (AI) has brought revolutionary changes in various fields, and its influence on medicine is particularly profound. AI is revolutionizing healthcare delivery, from diagnostics to treatments and drug discovery, like never before.
Medical image analysis is one of the primary fields where artificial intelligence has advanced significantly, and image labeling and annotation are essential. Additionally, research indicates that the global market for artificial intelligence in healthcare was estimated to be worth $4.9 billion in 2020 and is projected to grow at a compound annual growth rate of 44.9% to reach $45.2 billion by 2026.
This article will give you insights into the intricate workings of AI-driven medical image annotation, including its importance, challenges, and how it fundamentally changes the landscape of the healthcare field.
Table of Contents:
- Understanding AI in Medicine
- AI in Image Tagging and Annotation
- The Impact: How Medical Image Tagging & Annotation Powers Innovation
- Future Directions and Opportunities
- Final Thoughts
Understanding AI in Medicine
Before discussing the details of medical image tagging and annotation, it is important to understand what artificial intelligence (AI) in medicine means. AI is the simulation of human intelligence processes by machines, specifically computer systems.
In healthcare, AI algorithms are trained to analyze complex medical data such as images, patient records, or genetic information to help healthcare providers make decisions. Medical diagnostic imaging (AI) is key to diagnosing several diseases and conditions, ranging from identifying fractures in bones to detecting tumors in organs.
Nevertheless, interpreting medical images may be time-consuming and likely involve errors that require careful attention to detail. This is where AI-powered image tagging and annotation come into play, creating a revolutionary way out by simplifying this process and thereby improving diagnostic accuracy.
However, what do we mean when we talk about image tagging and annotation? Usually, a medical image, such as an organ part, abnormality, or anatomical structure, will be described within an image tag.
Likewise, annotation refers to giving more details or notes about these already labeled areas so that they can provide context and other information while interpreting them.
Also Read: How Can Gamification Be Used in the Healthcare Industry?
AI in Image Tagging and Annotation
Intricate machine learning algorithms drive advanced artificial intelligence-based image tagging and annotation. These algorithms are being trained on massive datasets that contain labeled medical images created through human experts’ input.
Moreover, in a method called supervised learning, AI can detect patterns and attributes present in such images, hence helping it accurately identify different structures and abnormalities.
However, the real magic happens when these models learned through AI are incorporated into real-life situations. When presented with new unlabeled medical images, the AI algorithm examines each pixel of the image, comparing them to the existing data for features it was trained on.
After identification, these features are assigned tags or annotated in a way that can be helpful to healthcare practitioners.
The Impact: How Medical Image Tagging & Annotation Powers Innovation
The adoption of AI in medical imaging is steadily increasing across healthcare institutions worldwide. According to a study, 83% of healthcare providers have either deployed or are planning to deploy healthcare AI applications within the next two years.
Besides, the integration of AI in image tagging and annotation has had a great impact on the field of medicine, changing how diseases are diagnosed and treated completely. These include:
- Faster Diagnoses: AI shortens diagnosis time by automating image interpretation, enabling healthcare providers to make timely treatment decisions. This is especially crucial during emergencies, where every second counts.
- Improved Accuracy: Human interpretation of medical images is subjective by nature and prone to errors. However, AI algorithms provide consistent and objective analysis, which leads to increased diagnostic accuracy and fewer missed diagnoses.
- Personalized Medicine: Through AI-powered image analytics, healthcare providers can design treatments based on individual patients’ unique attributes and disease profiles. This approach can potentially reduce the number of adverse drug reactions and improve outcomes.
- Enhanced Workflow Efficiency: When machines perform routine activities such as image tagging and annotation, medical practitioners have more time to spend on difficult areas of caregiving. This improves efficiency and mitigates stress levels among health workers.
Future Directions and Opportunities
The future of AI and machine learning in healthcare and medical image tagging and annotation holds immense promise. Here are some key areas of opportunity:
- Deep Learning Progress: The continued progress in deep learning algorithms, especially convolutional neural networks (CNNs), will further improve the accuracy and speed at which medical images are analyzed. This area will experience significant leaps forward with new architectures and training strategies tailored to medical imaging data.
- Multi-Modal Data Integration: Information from multiple imaging modalities like MRI, CT, or PET scans, clinical data, genomic information, and patient outcomes can help develop a more complete description of diseases. New AI algorithms that can process multi-modal data may offer new insights into disease mechanisms and treatment responses.
- Collaborative Research and Data Sharing: Researchers should collaborate with healthcare institutions and technology companies to fast-track building and validating AI algorithms, for example, in medical imaging. Innovations in medical technology, like open-access image repositories and collaborative research networks, promote these initiatives, resulting in creativity toward AI adoption in everyday clinical care.
Also Read: Slicing the Importance of Digital Accessibility in the Healthcare Industry
Final Thoughts
The secret sauce of AI in medicine is the ability of medical picture tagging and annotation to extract insightful information from intricate medical images. Furthermore, by utilizing annotated datasets and cutting-edge AI algorithms, healthcare providers can improve patient outcomes, individualized treatment planning, and diagnostic precision.
Despite the challenges and limitations, there is immense potential for imaging artificial intelligence in medicine. Ongoing developments will change healthcare delivery as we know it and bring about a new era of precision medicine.
Hurix Digital provides cutting-edge AI-powered healthcare solutions customized to your unique needs, whether you’re a healthcare facility trying to improve diagnostic accuracy and workflow efficiency or a research group trying to uncover new insights into disease causes.
With a proven track record of success and a commitment to excellence, Hurix Digital empowers healthcare providers and researchers to leverage AI’s maximum potential to transform the practice of medicine.
To learn more, contact us today!
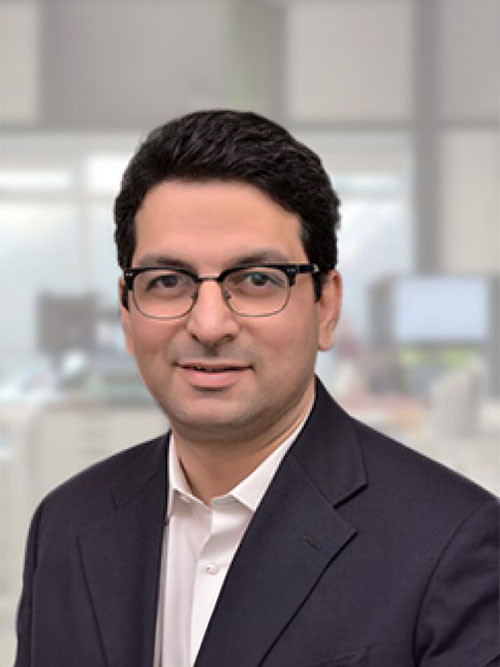
Senior Vice President
A Business Development professional with >20 years of experience with strong capability to sell new solutions and develop new markets from scratch. New Market Entry Specialist with experience of working in two of the largest emerging markets – China & India. Also covered other key markets in APAC, US, EU & ME. Exceptional experience of conceptualizing, ideating and selling new learning technologies like VR AR, etc. across multiple industry verticals.