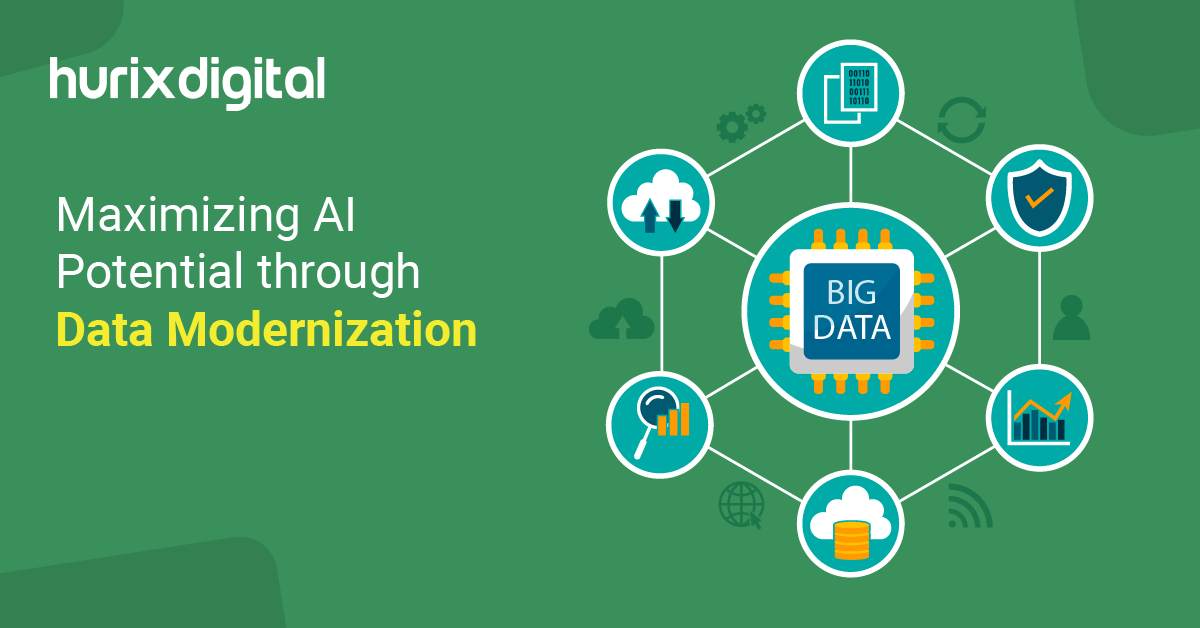
Maximizing AI Potential through Data Modernization
Summary
This article discusses data modernization as a foundation for AI analytics, addressing data challenges and outlining strategy benefits for AI accuracy.
Every business values information as an asset that requires handling. With businesses collecting an increasing volume of data, analyzing this data effectively is essential for gaining an edge.
Experts predict that the world’s data production will skyrocket to over 180 Zettabytes per year by 2025, underscoring the volume of data that companies must effectively handle and utilize.
The blending of intelligence (AI) and machine learning (ML) tools has transformed data analysis by revealing undiscovered patterns. Yet, for organizations to truly maximize the benefits of AI-powered analytics, they must initially lay a groundwork by modernizing their data systems.
Table of Contents:
- What is Data Modernization?
- The Rise of AI-Based Analytics
- The Data Modernization Challenges
- Building a Data Modernization Strategy
- Benefits of Data Modernization for AI Analytics
- Conclusion
What is Data Modernization?
Updating data systems to be more modern and adaptable is known as data modernization. This involves various tasks, such as;
Activity | Description |
Data Migration | Moving data from siloed systems and outdated formats to a centralized, cloud-based data lake or warehouse |
Data Cleansing | Identifying and correcting errors, inconsistencies, and missing values in the data |
Data Governance | Establishing policies and procedures to ensure the quality, security, and accessibility of data |
Data Integration | Connecting data from various sources to create a unified view of the information |
By implementing a data modernization strategy, organizations can reveal several benefits that are crucial for successful AI and ML adoption.
Also Read: Integrating Predictive Analytics and AI Into the Higher-Ed Curriculum
The Rise of AI-Based Analytics
AI-driven data analytics have emerged as a powerful tool for businesses across all industries. Foundry’s State of The CIO Study 2023 reports that application modernization remains the third-highest initiative for CIOs, highlighting its crucial role in driving business agility.
These technologies can be used for a wide range of applications, including:
- Customer segmentation and targeting
- Predictive maintenance
- Fraud detection
- Risk management
- Product development
By leveraging AI and ML, businesses can gain deeper insights from their data, identify new opportunities, and make data-driven decisions that improve their bottom line.
The Data Modernization Challenges
While AI-based analytics offer immense potential, their effectiveness hinges on the quality and accessibility of the underlying data. Unfortunately, many organizations struggle with data that is:
1. Data Silos and Inconsistency
Data is often siloed in different departments and systems, making it difficult to get a holistic view of the organization. Additionally, data inconsistencies and errors can lead to inaccurate results and misleading insights.
2. Outdated Infrastructure
Many businesses rely on outdated data infrastructure that is not equipped to handle the volume, variety, and velocity of modern data. This can lead to slow processing times and difficulty scaling to meet growing data needs.
3. Limited Data Governance
Without a strong data governance framework, organizations lack control over their data. This can lead to issues with data security, compliance, and overall data quality.
These challenges create a significant barrier to successful AI-driven analytics.
Building a Data Modernization Strategy
Here are some key considerations for building a data modernization strategy:
1. Assess Your Current Data Landscape
Start by conducting a thorough assessment of your current data landscape. This includes identifying your data sources, understanding how data is stored and managed, and evaluating data quality and consistency.
2. Define Your Data Goals
What do you want to achieve with AI-based analytics? Clearly define your data goals to guide your data modernization efforts.
3. Choose the Right Tools and Technologies
Numerous tools and technologies for modernizing data are at your disposal, including data lakes, data warehouses, and data governance platforms. Assess each based on your requirements. Choose the most suitable option.
4. Implement a Data Governance Framework
A strong framework helps in establishing trust that goes beyond technology. It defines policies, procedures, and roles for responsible data use, ensuring standards and processes for clean, consistent data for AI and ML. Assign clear ownership leading to data quality responsibility and define access controls to protect sensitive information.
Benefits of Data Modernization for AI Analytics
In the era of intelligence, data serves as the ingredient for driving discoveries and advancements. Similar to how a car requires fuel to operate, AI systems depend greatly on well-maintained, reliable, and readily available data to produce precise outcomes.
Enter data modernization – a process that involves revamping your data framework to unleash the capabilities of AI analysis.
Let’s explore the key benefits of data modernization brings to the table:
1. Improved Data Quality and Consistency
Consider training an AI model on data riddled with errors and inconsistencies. The results would be unreliable and misleading. Data modernization tackles this problem by cleaning and correcting errors in your data. This ensures AI ML solutions work with high-quality information, leading to more accurate and trustworthy results.
2. Enhanced Data Accessibility
In the past, information could be spread out in systems and fashioned structures. This created challenges for AI and machine learning programs to get the data for their training and growth. Data modernization gathers data into one platform, such as a data lake or warehouse. This simplifies access to data for AI programs, speeding up the process of creating, training, and implementing AI models.
3. Faster Time to Insights
Would you ever wait for weeks or months to get valuable insights from your data? Data modernization streamlines data management processes. By making data readily available and easier to analyze, AI can work its magic faster. This translates to quicker access to valuable insights that can inform critical business decisions.
4. More Accurate AI Models
The effectiveness of your data significantly influences the performance of your AI models. When your data is clean and consistent, AI algorithms can better grasp patterns and insights, leading to the development of dependable AI models that benefit businesses.
Also Read: A Brief Guide to How Predictive Analytics Boost Learning Results
Conclusion
The effectiveness of integrating AI as a tool greatly depends on the accuracy and reliability of the data utilized in the analysis. Data modernization serves as the bridge that transforms data into insights. Once the data modernization plan is put into action, you build the foundation for the future fueled with AI analytical tools.
This leads to countless advantages, which include customer satisfaction, organization performance, intelligent business procedures, and better decision-making.
Timely analysis and utilization of data should not be an unexplored possibility. Hurix Digital specializes in providing data—and AI-driven solutions for businesses.
Our team of analytics professionals can help you develop a data modernization strategy to unlock the potential of your data assets and align them with your organization’s objectives through the power of AI. Contact us today to schedule a consultation session.
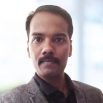
Currently serving as the Vice President of Technology Delivery Operations at HurixDigital, a prominent global provider of digital content and technology solutions for publishers, corporations, and educational institutions. With over 16 years of experience spanning EdTech and various domains, I hold certification as a SCRUM Product Owner (CSPO). My expertise includes operations, finance, and adept people management skills.