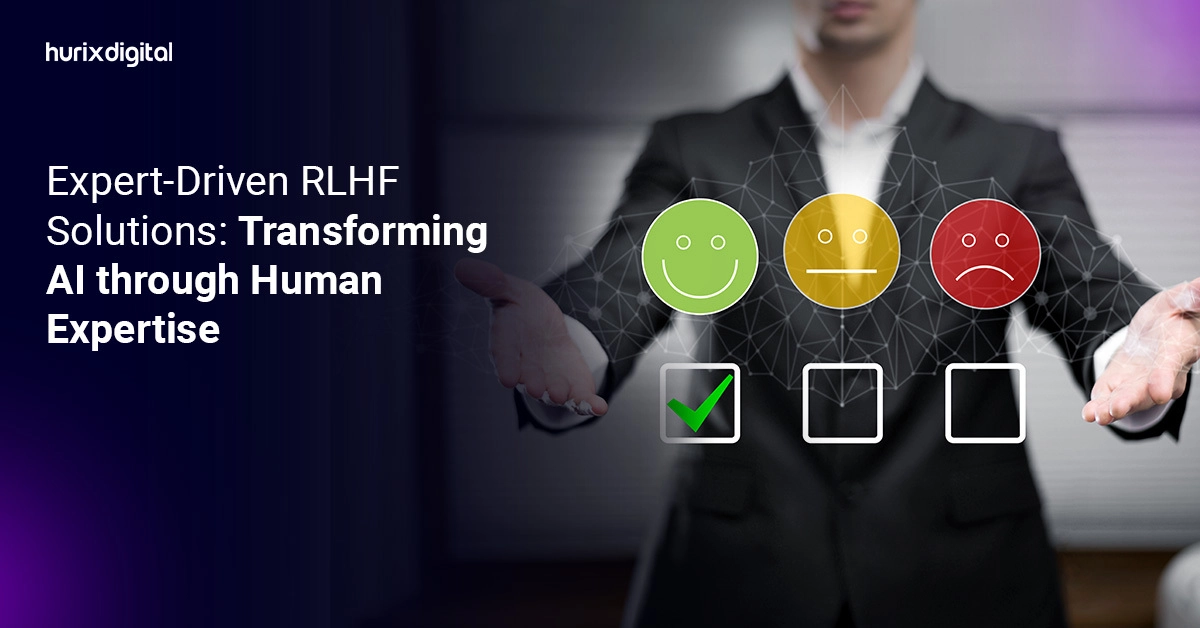
Expert-Driven RLHF Solutions: Transforming AI through Human Expertise
Summary
This blog explores expert-driven RLHF, where expert feedback guides AI systems to perform efficiently and ethically. It details RLHF’s workings, applications, benefits, and role in ethical AI development.
Artificial Intelligence (AI) is advancing rapidly, but aligning machine actions with human values remains a challenge. RLHF (Reinforcement Learning from Human Feedback) addresses this by integrating expert human feedback to train AI systems and also ensures that these systems perform efficiently and act ethically according to human expectations.
This blog explores the concept of Expert-Driven RLHF, its functions, applications, challenges, and benefits. We also highlight its role in shaping AI’s future and the importance of expert feedback for ethical AI development through deep reinforcement learning.
Table of Contents:
- What is Reinforcement Learning from Human Feedback (RLHF)?
- Expert-Driven RLHF: Why Experts Matter
- Benefits of Expert-Driven Approaches
- How Does Expert-Driven RLHF Work?
- Applications of Expert-Driven RLHF Solutions
- Benefits and Challenges of Expert-Driven RLHF
- The Future of Expert-Driven RLHF
- Conclusion
What is Reinforcement Learning from Human Feedback (RLHF)?
1. Reinforcement Learning Overview
Before we get into RLHF, it is necessary to understand the important concepts of AI Reinforcement Learning (RL). In RL, an AI learns by interacting with its environment and receiving rewards (positive reinforcement) or penalties (negative reinforcement) depending on the actions taken. The goal is to maximize total rewards over time, similar to human trial-and-error learning. However, traditional RL uses a fixed reward structure, which may not always align with human preferences and ethics.
2. Incorporating Human Feedback
RLHF includes human feedback to lead the learning process rather than simply depending on static reward signals from the environment. By directly influencing rewards, humans help the AI align its actions with its expectations and preferences. The system adjusts its behavior based on this human assessment.
Also Read: The Critical Role of Content Creation Experts in AI Video Tagging
Expert-Driven RLHF: Why Experts Matter
In expert-driven RLHF, subject matter experts provide feedback during AI training rather than random individuals. Their deep understanding of task complexities ensures precise and detailed guidance. For example, a doctor’s feedback is crucial for training AI in medical diagnosis, just as expert drivers’ input is vital for developing AI to drive.
Benefits of Expert-Driven Approaches
- Greater Precision and Consistency: Experts provide more accurate and adaptive feedback, which aids AI in performing effective studies.
- Ethical Consistency: With experts understanding ethical considerations and technical aspects, AI systems could be trained to function on moral values.
- Reduced Bias: Expert feedback helps reduce biases in training data, leading to more balanced and equitable AI systems.
- Quick Learning: Expert feedback is often more specific and practical, allowing AI systems to learn productively and achieve ideal performance faster.
How Does Expert-Driven RLHF Work?
Step 1: Initial Training Phase
In the beginning, the AI agent is trained using traditional reinforcement approaches. It engages with its surroundings, receives feedback through rewards or penalties, and incrementally learns to enhance its performance. However, at this stage, the reward structure may be elementary and insufficient to fully represent the complex criteria humans would use to assess success.
Step 2: Incorporating Human Feedback
After AI reaches a specific competency, human feedback provided by subject matter experts is introduced. This feedback is used to alter the reward signals, allowing the AI to modify its behavior in response to the expert’s input.
Step 3: Continuous Improvement
The process of receiving and acting upon expert assessment is continuous. As AI learns and improves, it gets better at meeting expert standards. Over time, the AI becomes highly skilled at adjusting its behavior based on environmental rewards and expert feedback.
Applications of Expert-Driven RLHF Solutions
Expert-driven RLHF solutions are used in diverse fields, from healthcare to autonomous systems. Here are some crucial areas having a considerable impact from this approach:
1. Healthcare
AI systems assist doctors not only in diagnosing and recommending treatments but also in performing surgeries. Expert-driven RLHF is crucial in these high-stakes applications, ensuring AI decisions are safe and ethical through expert feedback. For example, while AI may easily detect obvious cancerous lesions, it might struggle with subtle cases. Feedback from radiologists helps the AI learn to recognize these patterns missed during its initial training.
2. Autonomous Vehicles
Expert-driven RLHF is also vital in self-driving technology. Autonomous vehicles face complex environments and must make split-second decisions while prioritizing safety. By incorporating feedback from experienced drivers and engineers, AI systems learn to handle challenging driving situations effectively. This guidance helps the AI focus on actions that minimize risk and ensure passenger safety.
3. Content Moderation and Recommendation Systems
AI systems are responsible for distinguishing between harmful and benign content in moderation. This is a challenging task because the boundaries of what is considered damaging are unpredictable and can vary depending on the situation. By incorporating feedback from subject matter experts and ethical reviewers, AI can better align its moderation decisions with societal standards and human values.
Also, recommendation systems like streaming services and e-commerce platforms benefit from expert-driven RLHF. Experts in psychology and user behavior can provide relevant, helpful, and ethical recommendations.
4. Robotics
Expert-driven RLHF is also applied in robotics. Expert feedback ensures that robots are safe, effective, and meet human expectations, whether performing manufacturing tasks or caring for elderly individuals.
Benefits and Challenges of Expert-Driven RLHF
Benefits
- Greater Precision: Experts are involved in training AI systems to produce quality output. The detailed feedback experts provide helps fine-tune the AI system’s behavior.
- Ethical Consistency: Experts understand ethical considerations in addition to being technically proficient. This ensures that AI systems perform both effectively and morally acceptable.
- Reduced Bias: Experts can help identify and rectify AI output biases. Feedback from a knowledgeable and balanced perspective assists AI in making balanced and unbiased decisions.
- Faster Learning: Expert provides more precise and well-defined feedback, which aids AI in faster learning than depending entirely on environmental rewards.
Challenges
- Scalability: While expert feedback is incredibly valuable, it’s also difficult to obtain and expensive. Also, involving human experts at every learning stage for large-scale AI systems might not be possible.
- Subjectivity: Expert opinion is still biased even though it is more trustworthy than non-expert opinion. Differing expert opinions regarding the optimal course of action could result in inconsistencies in AI learning.
- Resource-Intensive: Incorporating expert feedback requires remarkable resources. These include time, money, and access to skilled professionals who can provide guidance.
The Future of Expert-Driven RLHF
The need for expert human feedback will grow as AI continues to evolve. Researchers are working towards creating fully autonomous AI systems, and expert-driven RLHF will play a crucial role in this development. Expert feedback ensures that AI systems operate efficiently while aligning with moral principles, reducing bias, and making ethical decisions.
Incorporating expert feedback into AI training will become increasingly important. For example, advancements in natural language processing will allow experts to provide more detailed feedback. As AI updates and advances, expert feedback from various fields will enhance the AI’s learning efficiency and improvement.
Also Read: 5 Ways AI Alt Text Generator Helps You Achieve WCAG Compliance Effortlessly
Conclusion
Expert-driven RLHF solutions present a crucial progression in AI technology. Incorporating human specialists’ knowledge and skills allows AI systems to execute tasks with greater accuracy, ethical consideration, and efficiency. Although issues such as scalability and subjectivity persist, the advantages of this approach are evident. AI systems are not only technically proficient but also aligned with human values and capable of making ethical decisions.
As AI’s role in our lives increases, expert-guided RLHF will ensure these systems operate safely, effectively, and ethically.
Transform your AI visions into reality with Hurix Digital, the foremost expert in RLHF and AI content services. Leverage our extensive experience with top publishers and mastery of AI learning to expedite the deployment of your AI agents. Choose Hurix Digital as your trusted partner—reach out now to get started!
Connect with us today to learn more about our AI content services.
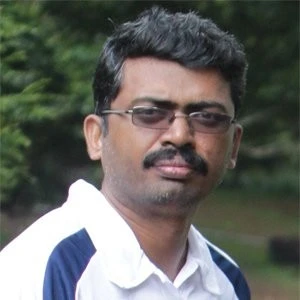
Vice President – Digital Content Transformation. He is PMP, CSM, and CPACC certified and has 20+ years of experience in Project Management, Delivery Management, and managing the Offshore Development Centre (ODC).