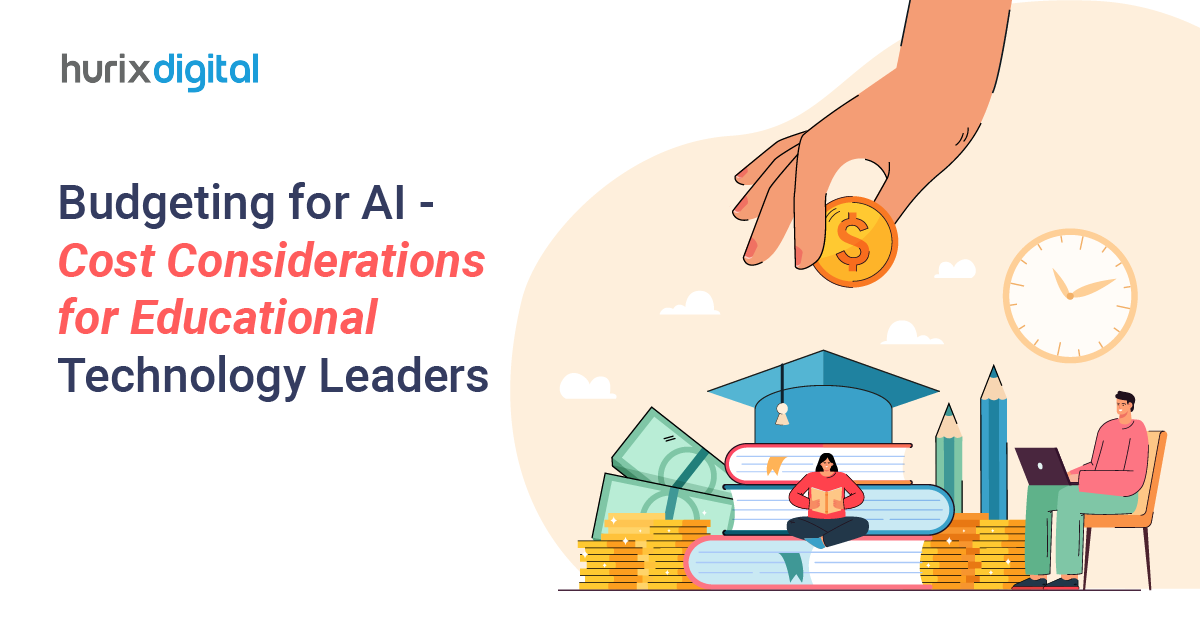
Budgeting for AI – Cost Considerations for Educational Technology Leaders
Summary
This article examines AI integration costs in education, covering hardware, software, data, maintenance, training, energy, budgeting strategies, cost-benefit analysis, ROI, and contingency planning.
One of the most important decisions educational institutions have to make in the era of AI is how much to invest in this transformative technology. The global EdTech budget for AI is predicted to shoot up to about $6 billion by 2025. Thus, it is no surprise that the AI revolution is steadily sweeping over education and creating some exciting new possibilities. One-size-fits-all teaching methods can be brought closer to AI for personalized learning, efficient handling of administrative tasks, and greater student success.
Unfortunately, such AI applications are not free. There is a cost associated with AI that educational institutions and EdTech leaders must understand before they can dip into the tech. Let’s explore the major cost factors, educational technology investments, and strategies that will help you budget for AI in your academic setting.
Table of Contents:
- Understanding AI Costs
- Key Factors Influencing AI Costs
- EdTech Budgeting Strategies for AI
- Wrapping Up
Understanding AI Costs
Integrating AI in education requires careful attention to cost components, distinguishing between AI-specific and traditional expenses like hardware and training. Scaling AI systems is essential; schools often start small but must accommodate more users and new features over time.
Plus, effectively managing AI EdTech budgeting requires a proactive approach that balances innovation with fiscal responsibility. Schools must budget for regular upgrades as AI technologies evolve rapidly. Maintaining efficacy is crucial in accommodating larger student populations or implementing AI-driven learning solutions across departments. This calls for careful long-term planning costs to avoid unexpected EdTech budgeting strain.
1. Hardware and Infrastructure
Complex AI will require hardcore servers with powerful CPUs, GPUs, and sufficient RAM. Large datasets and models, as well as the outcome of training processes, must be stored. A complex network infrastructure is required to handle high-speed data transfer and communication between AI components, which is often quite costly to maintain.
2. Software and Licensing
The most popular frameworks for developing AI EdTech models include TensorFlow, PyTorch, and Keras. Other popular libraries that offer pre-built algorithms and tools are available for a variety of machine learning tasks, such as sci-kit-learn, OpenCV, and NLTK. Data analysis, visualization, and model development using Jupyter Notebook, RStudio, or MATLAB are possible.
Yet, all these AI frameworks and tools have licensing and usage fees, which must be factored into EdTech budgeting.
3. Data Acquisition and Preparation
The best way to develop an AI system is to gather quality data. This could be done internally, through data acquisition partnerships, or sourced from external databases. Data cleaning and preparation tasks include handling missing values, removing outliers, and formatting the data to suit AI algorithms.
Supervised learning of data, whereby this data is labeled with correct outputs, is necessary to train the AI EdTech model. These costs can be detrimental to your EdTech budgeting, depending on the complexity of the task.
4. Maintenance and Updates
Server, storage system maintenance, and network infrastructure requirements have been updated regularly so that the AI EdTech program can perform best and be reliable. Incorporating these updated versions, which offer new features, bug fixes, and performance improvements, can increase your AI implementation costs.
5. Training and Development
Developing and maintaining AI systems by hiring or training skilled AI professionals, such as data scientists, machine learning engineers, or AI researchers, is essential. As a result, training AI models to meet specific requirements costs a lot, depending on the task’s complexity, the dataset’s size, and the hardware.
6. Energy Consumption
AI systems, especially those with powerful GPUs, can consume significant amounts of energy. This can impact energy costs and environmental sustainability. While choosing energy-efficient hardware and optimizing AI algorithms can help reduce energy consumption, they can further drive up expenses.
Also Read: Why Adopting WCAG 2.1 is Crucial for EdTech Companies: Pros and Cons
Key Factors Influencing AI Costs
As AI takes up an increasingly consequential role in the educational sector, assessing its financial implications is a factor to consider. A report estimates that worldwide spending on education will reach around $7.3 trillion by 2025, with AI being one of the significant contributors to that figure. Some of the considerations that will play a role in EdTech budgeting include the following:
1. Level of AI Maturity
- The earlier the application of AI in a project, the more cost-effective it may be because some fewer redundant existing systems or processes would need integration.
- AI on existing systems might be more difficult to integrate or change and thus adds to the cost.
2. Scope and Complexity of AI
- AI can be less expensive in simpler applications such as routine process automation.
- AI for complex tasks in the education sector and learning and development normally requires more complex algorithms, much larger datasets, and handcrafted hardware, which again increases the cost.
3. Data Volume and Quality
- The volume of the data will also determine the cost of training AI models. The more extensive the data, the more resources are required to handle it for either storage or computation.
- High-quality data is vital for good AI models. Poor quality data can lead to high costs from additional data cleaning, labeling, and retraining of the model.
4. Employees vs. Outsourcing to Third Parties
- It would mean significant upfront costs in hiring talent, acquiring hardware, and building infrastructure. On the other hand, internal development could provide much more control over the entire development process and ensure long-term ownership.
- Outsourcing AI development to vendors would lessen significant upfront costs and help tap some particular expertise. However, in the long term, this might also involve maintaining licensing fees or vendor lock-in.
5. Scalability and Future Growth
- The AI systems must scale with increases in data volume or user demands. Scalability can also affect costs, requiring more hardware, software, or personnel.
- While deciding on EdTech budgeting for AI investment, future growth, and potential expansion should be considered to avoid costly redesigns or re-implementations.
EdTech Budgeting Strategies for AI
For educational leaders, EdTech budgeting for AI is about grasping the technology and future-proofing student learning environments. Schools and other academic institutions need to plan strategically to maximize learning outcomes and operational efficiency.
1. Top-Down vs. Bottom-Up Strategies
- In the top-down approach, senior management decides on a fixed AI budget, after which the departments or projects bid for the allocated amount. This approach is efficient but generally does not require specific AI needs.
- The bottom-up approach has individual departments or projects determine their AI requirements and estimate the costs. The estimates are then aggregated to establish the overall AI EdTech budgeting expenditures. This approach may provide a more realistic view of the AI requirements but is much more resource-intensive.
2. AI Cost-Benefit Analysis
- Clearly define the expected benefits from AI EdTech use, i.e., greater efficiency, lower costs, or improved decision-making.
- Assign monetary values to these benefits, perhaps in terms of increased revenue, cost reductions, or improved student and administrative satisfaction.
- Another method for smart EdTech budgeting is to compare the initial and ongoing costs of AI with the estimated benefits to analyze the positive economic value of the investment.
3. Return on Investment for AI Calculations
- Divide the net profit from AI implementation by the total investment to get the ROI on your EdTech budgeting. A positive ROI means your investment is profitable.
- Consider the time value of money when calculating ROI because the benefits you receive in the future will be less valuable than those received today.
- Conduct sensitivity analysis to see how changes in different variables, such as costs, benefits, and time horizons, would affect the ROI.
4. Scenario Planning and Contingency Funds
- Develop different scenarios of possible outcomes, for example, best-case, worst-case, and base scenario.
- Estimate the cost in every scenario associated with hardware, software, people, and data gathering.
- Allocate a portion of your AI EdTech budget to contingency funds, which you would use to cover unexpected costs or challenges.
- Frequently monitor the AI EdTech project’s progress and make budgetary or contingency fund changes as appropriate to ensure successful implementation.
Also Read: 6 Best EdTech Content Accessibility Tips You Must Follow
Wrapping Up
EdTech budgeting for AI involves careful strategies and review. Education leaders must strategically use financial decision-making toward institutional goals, improve learning outcomes, and prepare the schools for shifting demands and roles amid an AI-driven environment.
While the integration of AI is expensive at initial investment, the costs for long-term benefits are usually offset. The sustainability of integrating it must take into account training, software updates, and AI maintenance costs, among others.
At Hurix Digital, we offer personalized EdTech budgeting solutions that will help you unravel the do’s and don’ts of AI implementation and optimize your EdTech investments.
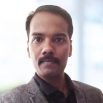
Currently serving as the Vice President of Technology Delivery Operations at HurixDigital, a prominent global provider of digital content and technology solutions for publishers, corporations, and educational institutions. With over 16 years of experience spanning EdTech and various domains, I hold certification as a SCRUM Product Owner (CSPO). My expertise includes operations, finance, and adept people management skills.