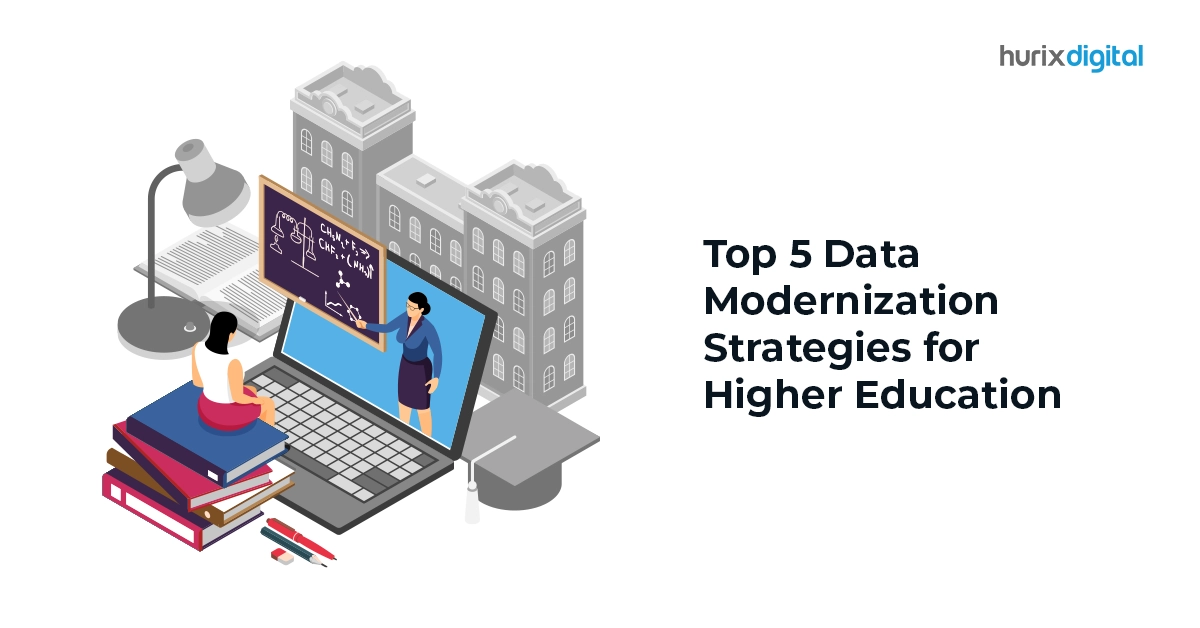
Top 5 Data Modernization Strategies for Higher Education
Summary
“Success occurs when opportunity meets preparation.” – Zig Ziglar
This concept is particularly relevant to the transformative potential of AI in higher education. Modernizing educational technology for teaching and learning centers on enhancing data compliance, architecture, integration, accessibility, and analytics.
Today, data modernization is crucial for businesses because it helps them in decision-making and operational efficiency. In fact, studies show that every day, almost 2.5 quintillion bytes worth of data is generated.
Using advanced techniques using Machine Learning and data analytics modernization, organizations, especially the education sector, can make the most of the full potential of their data.
Let us understand data modernization’s meaning and explore the top strategies that should be incorporated in higher education environments!
Table of Contents:
- Data Modernization: Meaning and Relevance in Higher Education
- Benefits of Data Modernization
- Top 5 Data Modernization Strategies for Higher Education
- Data Modernization Mistakes to Avoid
- Wrapping Up
Data Modernization: Meaning and Relevance in Higher Education
Data modernization is the process of upgrading from outdated databases to modern ones.
Data analytics modernization services help in managing various types of data and include cloud data warehouses which are more effective than traditional methods.
In fact, almost 74% of higher education schools were facing financial challenges in 2021. This is one of the main reasons why several of them have moved to data-modernizing strategies to combat hurdles.
Managing student data is a significant challenge for higher education institutions. Some of the key challenges are:
- Engaging prospective students
- Maintaining communication with current students and alumni
- Improving the use of data throughout the student lifecycle.
Today, it is important for colleges and universities to modernize data to enhance student engagement and outcomes.
Also Read: Exploring the Benefits of Text-to-Speech Technology in Educational Settings
Benefits of Data Modernization
Data modernization used in educational technology for teaching and learning provides a solution by developing systems and generating revenue. Here are several key benefits of data modernization:
1. Enhanced Data Accessibility
Data modernization breaks down barriers between different data sources. This makes it easier for educational leaders to access and utilize the necessary information across the organization.
2. Increased Flexibility and Efficiency
Modernized data infrastructure allows for faster data processing and efficient analysis. This enables universities and colleges to adapt quickly to market changes, student needs, and emerging opportunities.
3. Cost Efficiency
Data analytics modernization reduces costs by minimizing downtime and optimizing resource utilization. Investing in modern data solutions makes way for long-term sustainable growth and competitive market positioning.
4. Enhanced Security and Compliance
Modern data platforms offer advanced security features and compliance measures. This helps educational organizations protect sensitive data from threats and ensure adherence to regulatory requirements.
Top 5 Data Modernization Strategies for Higher Education
Here are the top 5 strategies that higher education organizations can use to encourage data modernization in educational technology for teaching and learning:
1. Identify and Prioritize Data for Cloud Migration
Higher education leaders need to prioritize data that encourages innovation and collaboration across departments. This ensures institute-wide connectivity and informed decision-making. Here are some tips:
- Develop a cloud-based data lakehouse to store structured, semi-structured, and unstructured data.
- Facilitate information flow and take advantage of the scalability and flexibility needed to handle massive data sets.
- Go beyond storing data by focusing on intelligent curation and utilization.
- Prioritize relevant and valuable data, employ advanced analytics, and utilize AI to extract actionable insights.
2. Ensure Effective Data Integration and Analysis
Form a collaborative team of data specialists, domain experts, and strategic planners skilled in cloud data management. Educational leaders can introduce the role of a Chief Data Officer, which is crucial for overseeing data integrations and analysis.
Here are some best practices to ensure effective data integration and analysis:
- Implement methodologies to optimize data workflows.
- Ensure your data warehouse and analytical tools are supported by the right talent.
- Centralize data assets
- Conduct a thorough inventory of your data assets.
- Format data according to the requirements of your AI models.
- Apply accurate labels and consider augmenting data to ensure a comprehensive dataset.
3. Ensure Data Quality
Focus on data quality by removing inaccuracies, handling missing values, and normalizing data. High-quality data is essential for AI models to detect patterns, predict outcomes, and provide actionable insights. Here are some simple suggestions:
Divide data into training, validation, and test sets to evaluate AI model performance effectively. Modernize your data by removing redundant, invalid, and inaccurate entries to ensure new data remains relevant. This is a critical step before undertaking any major data migrations.
- Develop a comprehensive governance framework to manage data governance, quality, and ethical use effectively.
- Make compliance with regulatory standards related to higher education norms that are central to this framework.
- Introduce auditing protocols to maintain data integrity. Integrate ethical sourcing and handling protocols into all higher education data practices.
- Quality assurance should be proactive, involving regular audits and feedback loops to address discrepancies and enhance data reliability.
- Engage educational leaders to encourage a collaborative and ethical data culture.
4. Integrate New Technologies with Existing Systems
Many higher education leaders struggle with integrating new data technologies with their existing systems. A balanced approach, considering both technological and human factors, can minimize operational disruption. Key integration goals include ensuring data compatibility and data security.
Your strategy should cover:
- Deciding whether to go for gradual integration or a complete overhaul
- Ensuring data compatibility with new technologies
- Training students and educators to work with both new and old systems
You can strengthen data security using:
- Comprehensive encryption strategies
- Zero-trust access management frameworks
- Continuous, real-time monitoring with immediate remediation
- Geolocated cloud mirroring
- Data backup solutions
5. AI and Data Modernization
Artificial Intelligence produces results from data inputs with limited user understanding of the process. Larger organizations establish AI centers of excellence to track, measure, and monitor AI initiatives.
Data quality is crucial, especially in data pipelines where poor data can affect multiple AI models. Implement an AI governance framework focusing on three key components:
- Data Lineage: Understand the origin of your educational data and its transformations over time.
- Data Scoring: Evaluate data quality based on profiling results and user rankings.
- Data Sharing: Once high-quality data is identified, make it accessible to other users through data marketplace tools.
Data Modernization Mistakes to Avoid
You must avoid these common pitfalls in data modernization:
1. Relying Too Heavily on Technology
Employing too many tools and vendors can increase data challenges or complicate quality measures. Integrating too many new tools with existing educational systems can be complex. It’s crucial to choose limited platforms and resources that can augment data utilization and modernize educational technology in higher education.
2. Not Having a Holistic Perspective and Focus
Data modernization initiatives often falter when they don’t align with the broader strategic objectives of the organization. Data modernization should be more than operational improvements and should transform the organization into a data-driven entity.
3. Treating Data Governance as a One-Time Project
Instead of treating data governance as a temporary concept, it is essential to adhere to best practices to ensure data reliability. This ensures that the data remains accurate, secure, and compliant over time.
Check out EXCLUSIVE: Hurix Digital Helps Prominent University Bridge the AI Knowledge Gap in Higher Education
Wrapping Up
Introducing data modernization in higher education institutions means focusing on data quality and integrating new technologies.
In this regard, you can collaborate with Hurix Digital to leverage AI and other advanced technologies. Our experts can help you develop and implement a robust data modernization strategy customized to your needs.
Get in touch with us today!
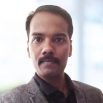
Currently serving as the Vice President of Technology Delivery Operations at HurixDigital, a prominent global provider of digital content and technology solutions for publishers, corporations, and educational institutions. With over 16 years of experience spanning EdTech and various domains, I hold certification as a SCRUM Product Owner (CSPO). My expertise includes operations, finance, and adept people management skills.